It’ll seem so obvious in 10 years, won’t it?
By 2034, Generative AI may well be on its way to Artificial General Intelligence, capable of outperforming humans at most economically valuable tasks. Possibly this AI boom will pop like another dot-com-style bubble, inflated by hype. Or maybe we’ll be living underground, relying on dogs to sniff out machine infiltrators.
Unfortunately, we’re stuck in the present: a time where AI conjecture, hype, and fear swirls around the public sphere. To cut through this noise, we in the ITX User Experience Content team determined there was only one remedy: the scientific method. We put ChatGPT-4 through its paces to understand if it’s something that can add value to our clients’ products. Here’s what we found.
Experiment 1: Is an AI Strategy Viable?
In our first experiment, we measured the impact on productivity and quality produced by integrating ChatGPT into our processes. Our hypothesis? That a UX Writer assisted by ChatGPT could produce publishable content faster than an equally skilled UX Writer – working without artificial assistance – with no discernible drop in quality.
We kept things simple by pitting two writers against each other, head-to-head. One could use ChatGPT as much as their heart desired, while the other followed our existing process. We gave them both a brief for a theoretical client’s product and sent them on their way.
The experiment’s results proved our hypothesis in a surprising way: our Large Language Model (LLM)-assisted writer produced the deliverable faster, and in surveys their work scored higher for quality. This result fascinated us and generated considerable discussion throughout the team and among our internal stakeholders, like:
- Could we discount the qualitative difference in skill levels between the experiment’s writers?
- If this tool is as powerful as the results suggest, should we prioritize replicating these results? Or should we move ahead with implementing the tool in our processes (and, if so, where in our processes?)
- What are the risks to ITX, our clients, and the products we co-create with them?
- What will our clients think?
Cautious optimism carried the day, but risk was a major topic of concerns. We determined that the best path forward would be to run a second experiment.
Experiment 2: Addressing AI Plagiarism and Accuracy Concerns
Our second foray into the world of LLMs for content creation focused on an issue of crucial importance to many peoples’ work across a range of industries: the risk of plagiarism and inaccuracies in LLM output. The risk that a given LLM may output factually incorrect – or stolen – information would weigh heavily on our decision to adopt these tools or avoid them until such risks decreased.
We aimed to test a process to check work for plagiarism and accuracy and determine if that added burden would diminish the productivity gains that the first experiment’s results suggested. In addition to our focus on the LLM’s integrity, we also sought to replicate the first experiment’s results.
Iterating on our method, we expanded our number of subjects from two UX Writers to three and assigned them a blog post for a theoretical client. One could only use ChatGPT to generate their post, the second could use ChatGPT as an assistant, and the third was prohibited from using any LLM assistance.
The results were exciting on two fronts.
1. We found no evidence of plagiarism or factual inaccuracy in any of the deliverables, and
2. We replicated the first experiment’s results when considering both quality and efficiency.
This time, we discovered some important nuance. Before we go there, though, let’s first focus on how we evaluate efficiency and quality. Not surprisingly, we measured efficiency based on the effort and time required to complete the task. As for quality, ITX team members across a range of disciplines rated the content’s quality on two criteria: how easy it was to engage with the content, and how easy was to understand
Now, let’s return to the important nuance. The post created by our writer with LLM assistance – that is, the work not completely delegated to the machine – scored the highest in terms of quality , but required the most time and effort to complete . The post generated fully by the AI scored the lowest for quality, but required the least amount of time to produce. Our human-generated control scored in the middle of these two extremes.
Results: Should we use LLMs in content work at ITX?
The results of these two experiments have convinced us that yes, using these tools is a net positive that will drive greater value for our clients.
However, the question of where in our processes we should use these tools remains unanswered. Our results prove that simply adopting an LLM and relying on it exclusively would be undesirable when quality and readability are essential requirements . Instead, we should identify those points in our processes where we can maximize the effect of the LLM.
Despite all the hype, it’s important to know that LLMs have limitations. We need to consider them and evaluate their potential impact as we determine where in our content development process we should apply these tools.
For example, limited context windows – that is, the number of tokens that an LLM can hold in its “memory” – render them ineffective at creating content from a long list of inputs and prompts. Experiment subjects reported frustrations with this.
“You refine prompts by asking [ChatGPT] to change three things, and it’ll change two of them. But then it completely forgets about the one from two prompts ago,” noted one UX Writer at ITX.
Insights: Where to Adopt LLMs Today
With these limitations in mind, we’ve identified two broad areas where we’re investigating adoption.
1: Rapid outlining
LLMs can help us rapidly generate an initial outline for a content deliverable. We can adapt to issues with the context length (for example, a writer needing to comb through dense outputs to catch issues or discover forgotten prompts) by asking the AI to produce short outputs, such as outlines and brainstorms.
LLMs excel particularly at summarizing sources like scientific papers; these summaries help the content writer efficiently gather information they need to begin their work.
Hallucinations remain a real danger, but human intervention in the form of an expert writer is reduces this risk; with a human still responsible for writing the piece and citing their sources, there is little risk of a hallucination worming its way into the final output.
2: First-pass reviews
People are busy, which means that one key bottleneck in our process hampering overall productivity is peer review. We believe that leveraging LLMs like ChatGPT-4 to perform an independent, “first pass” review can reduce the impact of this bottleneck. Because LLMs are good at analyzing segments of text that fall within their context window limits, the tool is ideally suited for this first pass task.
Take caution, as more investigation is required here. Adopting an LLM for this purpose often requires prompting an LLM with client information, so it’s imperative to address information security in a way that ensures that no NDAs are violated, and no confidential client data inadvertently makes its way into an LLM’s training data set.
What’s Next?
New technology can be intimidating. The experience of seeing ChatGPT stamp out lines of copy seemed to conjure a connection through time to those British weavers of the 1770s, who at the very dawn of the industrial era witnessed mechanized looms spin the textiles that had been the source of their livelihoods. We use the example of the Luddites intentionally, as history demonstrates that there’s no sense in fighting breakthroughs that have the potential to improve your ways of working.
In the UX Content team, we’ve all been working hard to identify ways to use this new technology to the advantage of our clients, to help their products perform more powerfully for a smaller investment. At ITX, we don’t blindly believe the hype; one of our core values is Innovation through Experimentation. We live this value by way of our commitment to testing new technologies, like large language models, that have the potential to move, touch, and inspire the world.
Heading
This is only a tiny fraction of our findings. To read our experiment results in detail, which includes discussion on the future of these Generative AI tools incorporating the cutting-edge research in this space, reach out today.
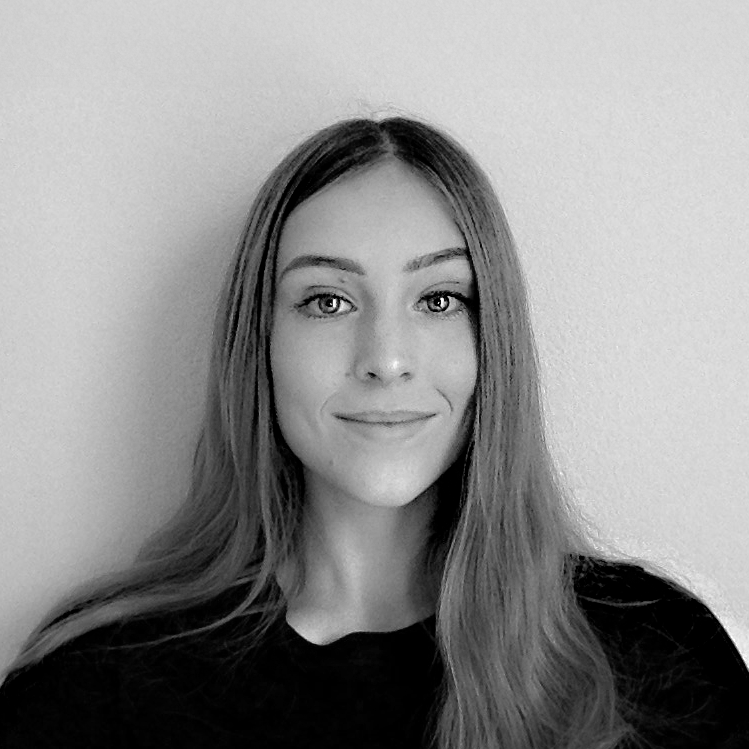
Lydia Pejovic is a UX Writer at ITX. She enjoys creating informative and engaging content for all audiences. Lydia received her BA in English from the University of San Diego and a dual MA & MFA in English from Chapman University.
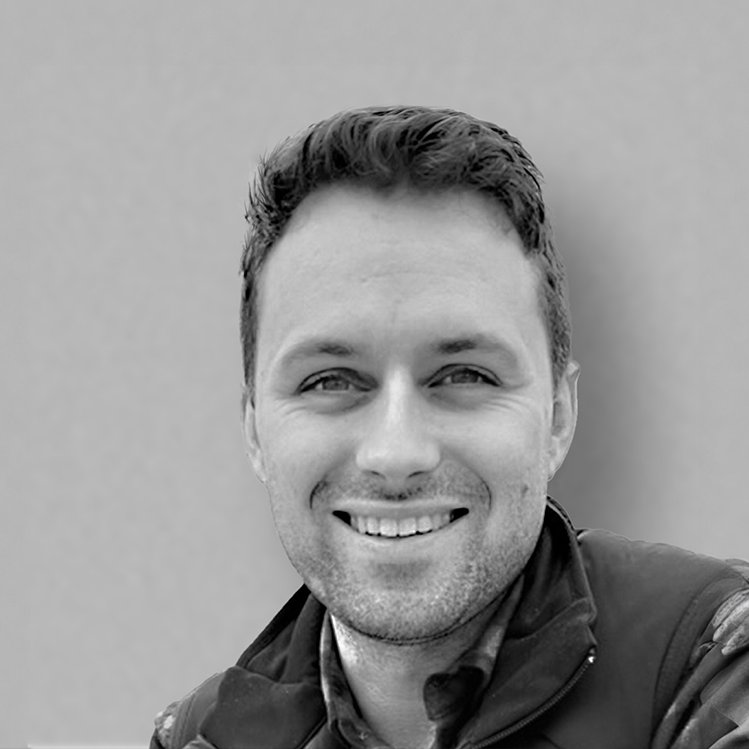
Tim Snedden leads the UX Writing and Content Strategy practice at ITX. His work revolves around the idea that excellent communication underpins every great digital experience.